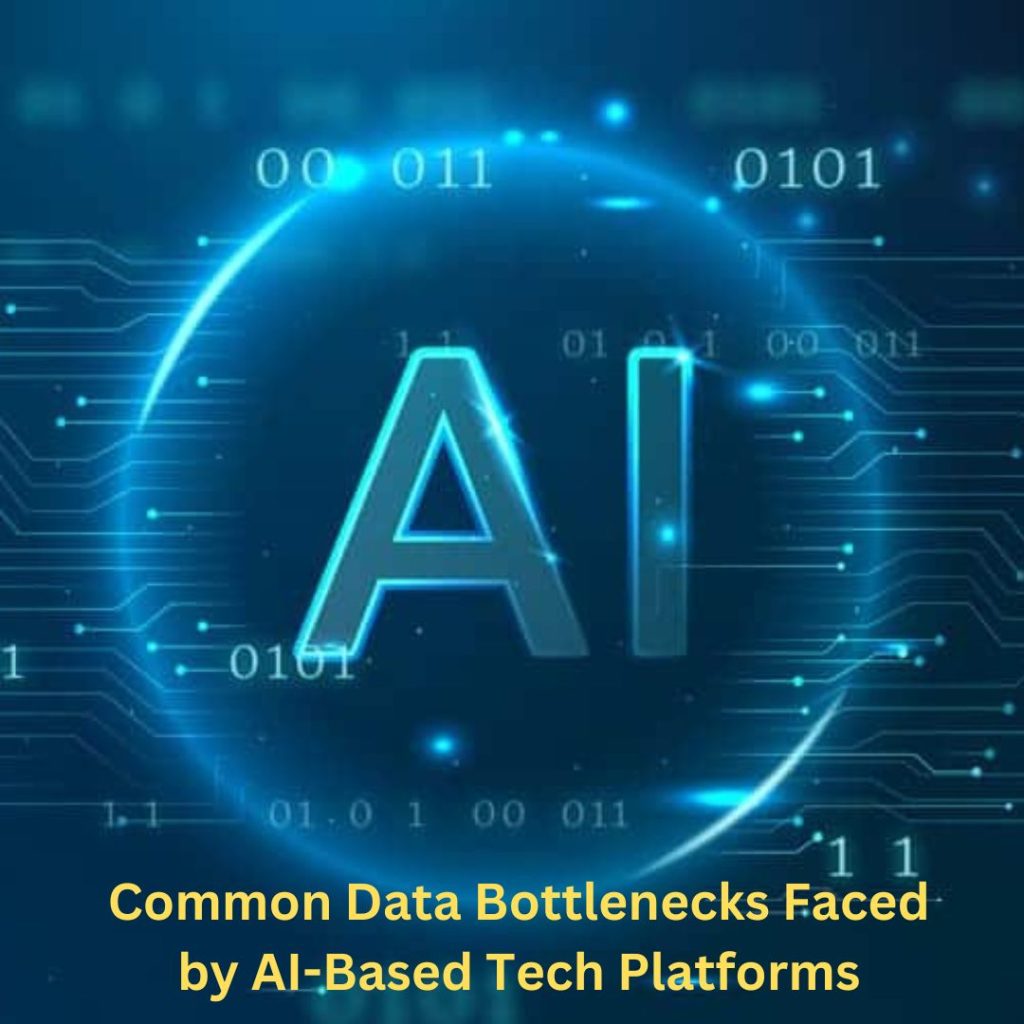
AI-based software and solutions are rapidly transforming various industries, from healthcare and finance to eCommerce and automotive. However, their success heavily relies on the quality of the data they utilize. These tech platforms utilize data at every stage of their operations, from training machine learning models to real-time inference and decision-making. These platforms ingest data from diverse sources, including user interactions, sensors, databases, and external feeds. As these platforms handle ever-increasing volumes of data, they often face various data-related challenges that can hinder their performance, accuracy, and scalability.
In this blog, we will explore the common data bottlenecks faced by AI-based tech platforms and provide solutions to overcome these challenges. By addressing these, organizations can improve their AI solutions while ensuring data quality, compliance, and operational efficiency.
Table of Contents
Addressing 4 Data Bottlenecks Faced by AI Platforms
1. Presence of Incomplete, Inconsistent, or Erroneous Data
Incomplete data can significantly distort the functioning of AI models, leading to biased or inaccurate predictions. When datasets have missing values, the models may not learn the true patterns or correlations, resulting in suboptimal performance. When data from different sources is in inconsistent or varying formats, it becomes challenging to align them into a cohesive structure that AI models can effectively learn from. Moreover, noisy or erroneous data, which can result from errors in data collection or entry, can skew the models’ outputs, leading to unreliable and misleading results.
Solutions: Addressing these issues involves using data imputation techniques such as mean or median substitution and regression-based methods to fill in missing values, ensuring a more complete dataset. Implementing data enrichment, data standardization, and data normalization processes maintains consistent data formats across various sources, facilitating easier integration and analysis. Additionally, outlier detection methods can identify and manage anomalous data points, while deduplication processes remove redundant or erroneous entries, ensuring the data used for training and operation is as accurate and clean as possible.
2. Data Integration and Management Challenges
Organizations often store data in separate, isolated systems, known as silos. This means that different departments or teams have their own data sets that are not shared or integrated with others. As a result, the organization has a fragmented understanding of its data because the data sets do not interact with each other. This fragmentation can lead to incomplete insights, making it difficult to make well-informed decisions.
Furthermore, the vast volumes and variety of data generated today can also strain traditional storage and processing systems, resulting in performance bottlenecks and resource constraints. Efficient data management is critical for extracting valuable insights and maintaining system performance.
Solutions: Data integration strategies such as ETL (Extract, Transform, Load) pipelines streamline the process of consolidating data from multiple sources into a unified view, enabling better analysis and insights. Data virtualization allows for real-time access and integration of data without the need for physical consolidation, enhancing flexibility and efficiency. Scalable storage solutions, such as cloud storage and distributed file systems, can handle large volumes of data effectively. Distributed data processing frameworks like Apache Spark and Apache Kafka facilitate efficient data processing and analysis, minimizing performance challenges.
3. Data Privacy and Security Concerns
Handling sensitive or personal data introduces significant compliance risks, as organizations must adhere to regulations such as GDPR or CCPA. These regulations mandate stringent data privacy and security measures to protect individuals’ information. The potential for data breaches not only jeopardizes individuals’ privacy but also exposes organizations to severe financial and reputational damage due to regulatory penalties.
Solutions: To protect sensitive information, data anonymization techniques can be applied, transforming identifiable data into a format that cannot be traced back to individuals. Robust access controls ensure that only authorized personnel can access sensitive data, while encryption mechanisms protect data both in transit and at rest. Establishing comprehensive data governance frameworks helps organizations manage compliance by defining policies and procedures for data handling, ensuring that all data activities are traceable and accountable.
4. Ongoing Data Quality Monitoring and Maintenance
One of the most common data bottlenecks faced by AI-based solutions is undetected data quality issues that can lead to model drift, where AI model performance degrades over time. Stale or outdated data can compromise the accuracy of AI models, leading to suboptimal predictions. Continuous monitoring and maintenance of data quality are essential to sustain the performance and reliability of AI systems.
Solutions: Implementing continuous data quality monitoring tools allows for real-time detection of data quality issues, enabling prompt corrective actions. Automated alerts can notify data managers of potential problems before they impact the models. Opting for B2B data cleansing can streamline the process of correcting and cleaning data, ensuring it remains accurate and reliable. Data enrichment strategies involve updating models with new and relevant data, keeping them current, and improving their predictive power.
A Comprehensive Solution to Resolving Data Challenges: Data Cleansing Services
Among all major data bottlenecks, the most critical challenge that AI-based solutions and software providers can encounter is maintaining data quality. Data quality issues can arise from various sources, such as human errors, inconsistent formatting, duplicates, missing values, and outdated information. Addressing these issues is crucial for organizations to extract meaningful insights and build robust AI solutions.
One effective approach to addressing data quality challenges is to opt for B2B data cleansing services. Data cleansing involves a range of processes aimed at enriching, normalizing, standardizing, deduplicating, and validating data to ensure its accuracy, consistency, and completeness.
Implementing an effective data cleansing strategy requires extensive resources and infrastructure. Service providers specializing in data cleansing have the necessary skills, tools, and processes to tackle these challenges efficiently. They often employ a human-in-the-loop approach, combining advanced automation techniques with human oversight and expertise. This approach ensures that while certain data tasks are automated for efficiency, a human perspective is maintained to address complex scenarios and edge cases that may require manual intervention.
To Conclude
Organizations aiming to implement successful AI projects must focus on getting rid of data bottlenecks. Overcoming these requires a concerted effort from organizations involving collaboration between data engineers, AI developers, business stakeholders, and data cleansing services providers. By adopting best practices, leveraging advanced technologies, and fostering a data-driven culture, AI-based tech platforms can effectively navigate these challenges and unlock the transformative potential of artificial intelligence.
More Stories
Coronavirus, a pandemic in times of Artificial Intelligence